The Evolution of CAD and AI: Reshaping Product Design in 2025
- Sarmad Sarwar
- Feb 2
- 6 min read
Updated: Feb 16
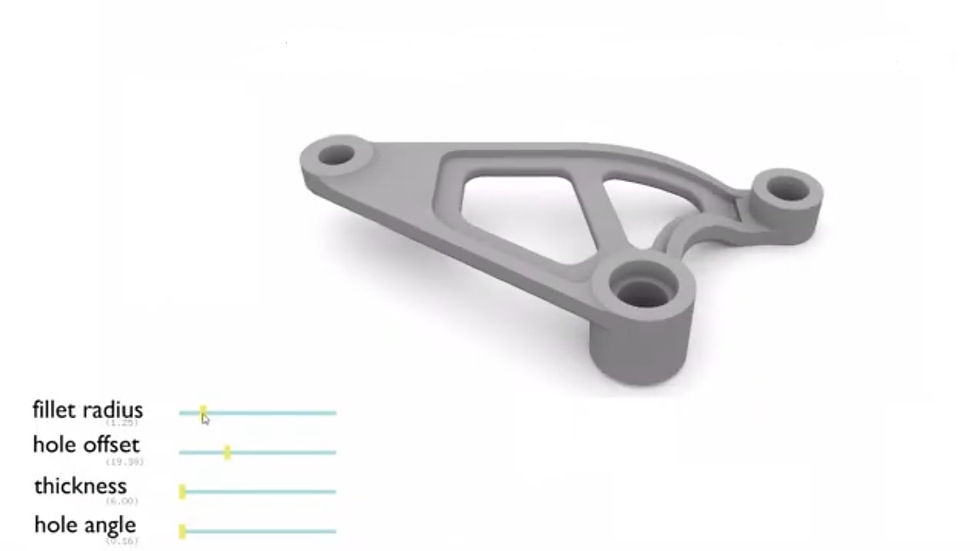
While everyone is talking about the infusion of AI into the CAD, I thought I should share my opinion, too. It's no longer a secret that Artificial intelligence (AI) is into everything and computer-aided design (CAD) is no exception.
The use of AI in CAD systems offers groundbreaking potential to streamline design processes, reduce human errors, and introduce innovative solutions to design challenges. Recently, platforms like AdamsCAD have begun exploring the synergy between AI and CAD, showcasing the promising future of intelligent design tools. This article delves into the various facets of AI integration into CAD, its impact on design automation, optimization, and analysis, the benefits and limitations it presents to designers, and the broader implications for the job market.
The integration of AI into CAD platforms can be seen as a natural evolution of design tools, enabling smarter, more efficient workflows. By embedding AI algorithms within CAD systems, software can leverage data-driven insights to guide design decisions. For instance, AI can assist in generating design suggestions based on historical data, simulate the impact of different design choices, and identify potential flaws before the design reaches the prototyping stage. Machine learning techniques can be used to train the software on vast datasets, allowing it to recognize patterns in designs that human designers may not immediately notice.
One notable example of AI in CAD is AdamsCAD, which has attempted to merge AI with its design software. This integration allows for faster design cycles and more accurate predictions in terms of material performance, structure, and even cost estimations. By analyzing the complexities of a design and its intended use, AI-driven systems can propose optimized solutions that reduce errors and costs while improving the overall functionality of a product.
AI's role in design automation could be profound, particularly in areas such as generative design and drafting. Generative design is an AI-powered process where the software automatically generates multiple design alternatives based on predefined parameters. These parameters could include material types, weight restrictions, and even cost limitations. The software then evaluates each design option, selecting the most efficient and viable choices based on performance criteria, enabling designers to choose the best solution quickly.
For example, Autodesk's generative design tools in CAD allow designers to input goals, such as strength, weight, and material requirements. The software then suggests various design alternatives, each optimized for the given parameters. The AI learns from previous designs and gradually improves its ability to produce optimal results. This not only accelerates the design process but also encourages creative exploration, as AI can uncover solutions that a human designer might not initially consider.
Drafting, too, benefits from AI-driven automation. By automating repetitive tasks such as aligning objects, adjusting dimensions, or checking for inconsistencies in a design, AI allows designers to focus on more strategic decisions. This leads to faster design iterations and fewer manual errors, ultimately improving productivity.
Topological optimization, a design process that optimizes the material distribution within a given space for a set of load conditions, is another area where AI has made significant strides. Traditionally, topological optimization has been a highly complex task requiring significant manual input and computational resources. AI can enhance this process by analyzing large datasets and identifying the most efficient material layout based on performance requirements.
AI-driven topological optimization tools can simulate a wide range of potential designs in a fraction of the time it would take a human designer. The AI models can analyze the interaction of forces on materials and structures, optimizing the design for maximum strength and efficiency while minimizing material waste. This has significant implications for industries such as aerospace, automotive, and construction, where weight reduction and material efficiency are critical.
Additionally, AI-powered simulations and analysis tools can predict the performance of a design under real-world conditions. By automating the simulation process, AI makes it easier to assess the viability of a design, forecast potential failures, and optimize for durability and performance. These AI-driven insights can save significant time and resources by identifying issues early in the design process.
For product designers and design engineers, AI integration into CAD platforms promises to ease many aspects of the design process. The automation of repetitive tasks, generation of design alternatives, and rapid analysis of simulations allows designers to spend less time on tedious work and more time on creative, high-level decision-making. AI can act as a co-designer, offering suggestions and optimizations that might not have been immediately apparent, thereby increasing both productivity and creativity.
However, despite these advantages, AI cannot replace the expertise of seasoned designers. While AI tools can assist with design generation and analysis, they lack the nuanced understanding of material properties, inherent material behaviors, manufacturing constraints, assembly processes, and aesthetic judgment that human designers bring to the table. AI might suggest a design that looks optimal on paper, but it may not account for practical challenges like manufacturability. Moreover, AI’s "black-box" nature can lead to situations where it makes decisions that are difficult for human users to interpret or explain, which can lead to significant errors if not carefully monitored.
Despite its capabilities, AI in CAD still would have limitations that will prevent it from completely replacing human expertise. One major limitation is that AI systems are heavily reliant on data. Without high-quality, diverse datasets, AI tools may not generate effective solutions, especially in unique or unfamiliar design contexts. Furthermore, while AI can suggest efficient designs based on historical data, it does not understand the real-world implications of a design as deeply as a human does.
Material properties and manufacturing processes are another area where AI falls short. While AI can optimize designs based on theoretical parameters, it may not always account for the nuanced characteristics of specific materials or the complexities of manufacturing processes. The knowledge of material behavior, machining techniques, and tolerances still requires the intuition and expertise of experienced engineers.
Another challenge is that AI systems are prone to making drastic mistakes, particularly in complex or unpredictable design scenarios. While AI can efficiently handle standard, well-understood design tasks, it may struggle with more intricate or innovative problems that demand human insight, critical thinking, and intuition. Designers and engineers must, therefore, remain engaged in the decision-making process to ensure the AI’s recommendations align with practical and contextual considerations.
As AI continues to integrate into CAD platforms, its impact on the job market remains a topic of debate. While AI promises to automate many aspects of design, it is unlikely to replace designers and engineers entirely. Instead, the role of the designer may evolve, with professionals focusing more on overseeing AI-driven processes and managing the creativity and strategy behind designs. In this sense, AI could free up time for more high-level, value-added work, but it could also lead to the displacement of tasks that were once manual.
Moreover, the need for AI experts in CAD will rise. As AI systems become more complex, companies will require skilled individuals who can develop, train, and manage these AI systems. This shift may create new opportunities in AI development and maintenance, but it will also require the workforce to adapt and learn new skills.
Two notable examples where AI has been used in design optimization highlight its potential. The first is the use of generative design by Boeing in the aerospace industry. Boeing has employed AI to generate lightweight components for aircraft, optimizing material usage while maintaining structural integrity. This has led to significant cost savings and improved performance, showing the power of AI in highly regulated and technical industries.
The second example comes from the automotive industry, where companies like General Motors have utilized AI in topological optimization. AI-driven software has helped GM optimize parts for weight reduction without compromising strength, thus improving fuel efficiency and reducing manufacturing costs.
AI integration into CAD has the potential to revolutionize the design process, offering enhanced automation, optimization, and analysis. While it can ease many aspects of design, from generative design to topological optimization, it is important to remember that AI is not a replacement for human expertise. The true value of AI lies in its ability to augment the capabilities of designers and engineers, offering them a powerful tool to streamline processes and explore creative possibilities. However, the complexities of design, especially in areas involving material properties and manufacturing constraints, still require human judgment. As AI in CAD continues to evolve, the role of the designer will shift, but the need for human expertise and oversight will remain indispensable.
Important Note: The subject and opinion of the article are of the writer's while some of the content was sourced and corrected by AI.
Comments